The venture capital industry is currently undergoing a significant transformation, propelled by the advent of advanced data analytics and machine learning technologies. This evolution transcends traditional quantitative analysis, delving deep into market trends, startup viability, and the underlying psychological factors influencing investment decisions. The crux of this article is to elucidate the multifaceted impact of data on the venture capital sector, providing a holistic view of contemporary VC strategies. As we navigate through this data-driven landscape, we’ll uncover the pivotal role that artificial intelligence, machine learning, and unconventional data sources play in refining investment processes, risk management, and portfolio optimization, marking a new era in venture capital decision-making.
The Emergence of Data-Driven Investments
Understanding the Role of AI and ML
Artificial Intelligence (AI) and Machine Learning (ML) are at the forefront of revolutionizing the venture capital ecosystem. These technologies offer predictive analytics capabilities that significantly enhance the identification of promising investment opportunities and the efficient management of portfolio risks. By leveraging AI and ML, venture capitalists can sift through extensive datasets to unearth trends and patterns not immediately apparent through traditional analysis methods, thus making more informed and strategic investment decisions.
The Power of Public Web Data
In the age of information, unconventional data sources such as public web data and satellite imagery have emerged as goldmines for venture capitalists seeking unique insights into potential investments. For example, analysis of parking lot traffic via satellite images has provided unexpected indicators of company performance, such as identifying a surge in customer visits to retail stores. This approach enables investors to stay ahead of the curve, capitalizing on opportunities that might have been overlooked using traditional investment models.
Risk Assessment and Market Prediction
From Early-Stage to Mass Market
Assessing the potential for success from an early stage through to mass market adoption involves intricate risk evaluation and market prediction methodologies. By applying frameworks adapted from Geoffrey Moore‘s work on crossing the chasm and achieving mass-market success, venture capitalists can evaluate startups’ potential to transition from niche markets to widespread acceptance. This process includes detailed risk assessments of market adoption, product viability, team capabilities, and financial stability, providing a comprehensive analysis of a startup’s journey to industry leadership.
Predictive Models in Action
Machine learning models are increasingly being utilized in high-frequency trading (HFT) and signal generation, showcasing the breadth of data analytics in venture capital. In HFT, AI’s capability to process vast amounts of data at incredible speeds allows for the execution of numerous orders in seconds, a feat unachievable by human traders. Moreover, ML algorithms play a crucial role in generating investment signals by analyzing a wide array of data, including historical price patterns, market sentiment, and volatility. These applications underscore the transformative impact of machine learning on venture capital, enabling more precise and timely investment decisions.
The integration of data-driven strategies in venture capital heralds a new era of investment decision-making. By harnessing the power of AI, ML, and unconventional data sources, venture capitalists are equipped to make more informed choices, manage risks more adeptly, and ultimately, achieve greater success in the competitive startup ecosystem.
Overcoming Human Limitations with Data
Balancing the Psychological Aspects
Venture Capitalists (VCs) are not immune to the psychological biases that affect us all. Two prevalent biases in the VC world are the Fear of Missing Out (FOMO) and the Fear of Looking Stupid (FOLS). FOMO can drive VCs to rush into investments for fear of missing the next big thing, while FOLS might cause them to pass on potentially groundbreaking opportunities to avoid the risk of failure. These biases can lead to decision-making that is not always rational or in the best interest of their portfolios. Data-driven decision-making can help balance these biases by providing objective insights and analyses that support or challenge investment theses, guiding VCs to make more informed decisions that are based on evidence rather than emotion or psychological biases.
The Importance of Founder Personality
The personality of a startup’s founder can significantly influence a VC’s investment decision, often more than the startup’s business plan or product. Founders who can strike a balance between fast, intuitive decision-making (System 1 thinking) and slower, more analytical reasoning (System 2 thinking) are particularly appealing to VCs. However, the reliance on “gut feelings” about a founder’s personality can introduce bias, such as the Mere Exposure Effect, where VCs may favor founders with similar backgrounds to their own. Incorporating data-driven approaches, such as using AI to analyze founder personalities or track record success, can provide a more objective basis for evaluating a founder’s potential to successfully lead a startup, thereby optimizing investment choices by complementing human judgment with hard data.
Leveraging Data for VC Success
A Step-by-Step Guide to Data-Driven VC
Integrating data analytics into the VC investment process can significantly enhance a firm’s ability to source deals, assess risks, and ultimately, make more successful investments. Here’s a practical approach:
- Data Collection: Start by gathering comprehensive data from a variety of sources, including public web data, financial records, and market analyses.
- Analytics Implementation: Utilize machine learning algorithms to process and analyze the collected data. This could involve predictive analytics to identify potential investment opportunities or risk assessment models to evaluate the viability of startups.
- Decision Support: Use the insights gained from data analytics as a basis for investment decisions, supplementing traditional methods and intuition with objective, data-driven analysis.
- Monitoring and Adjustment: Continuously monitor the performance of investments and the market landscape, using data analytics to identify when adjustments or interventions are needed.
Navigating Challenges
While the integration of data analytics offers numerous benefits to VCs, it also comes with its set of challenges. Issues with data quality can lead to inaccurate analyses, while an over-reliance on data can neglect the invaluable insights that human intuition and experience provide. To navigate these challenges, VCs should:
- Ensure access to high-quality, reliable data sources and implement rigorous data validation processes.
- Maintain a balanced approach that combines data-driven insights with the nuanced understanding and judgment that experienced investors bring to the table.
- Stay agile, being ready to adapt strategies based on new data or changes in the market environment.
Leveraging data for VC success involves a blend of sophisticated analytics and seasoned investment acumen. By adopting a data-driven approach while acknowledging and addressing its inherent challenges, VCs can enhance their decision-making processes, uncover new opportunities, and achieve greater success in the competitive landscape of venture capital.
AI and ML Transforming VC Landscape
Case Studies of Success
Venture capital firms are increasingly leveraging AI and ML to enhance their investment processes, with several notable successes already shaping the industry’s future. Correlation Ventures is a prime example, utilizing in-house ML models to analyze data from over 100,000 venture capital rounds. This data-driven approach enables them to make investment decisions in under two weeks, significantly faster than traditional methods. Similarly, EQT Ventures employs an AI framework named “Motherbrain” to guide its investment decisions based on proprietary data, startup financials, and more. This tool has allowed them to identify high-potential investments more efficiently.
Navigating the Future: AI and ML in Venture Capital
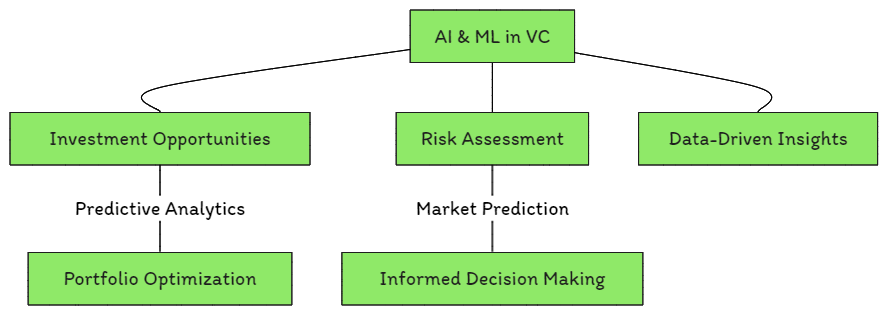
In the rapidly evolving landscape of venture capital, Artificial Intelligence (AI) and Machine Learning (ML) have emerged as pivotal forces driving innovation and strategic decision-making. This transformative shift is not merely a trend but a comprehensive overhaul of traditional methodologies, propelling the VC sector into a new era of data-driven investment strategies. As we delve into the core of this revolution, we uncover how AI and ML are redefining the processes of identifying lucrative investment opportunities, conducting thorough risk assessments, and harnessing data-driven insights to optimize portfolio performance. This article section aims to explore the nuanced role of AI and ML in enhancing the precision, efficiency, and success rates of venture capital endeavors, marking a significant leap towards a future where data reigns supreme in the quest for groundbreaking innovations and substantial returns.
Through this exploration, we witness the unparalleled capacity of AI and ML to sift through vast datasets, unveiling patterns and insights that elude traditional analysis. This capability allows venture capitalists to pinpoint startups with the highest potential for success and to tailor their investment approaches with unprecedented accuracy. Furthermore, by automating risk assessments and leveraging predictive analytics, VCs can navigate the complexities of the startup ecosystem with greater confidence, ensuring a more robust and resilient portfolio.
Moreover, the integration of AI and ML extends beyond mere number crunching; it introduces a paradigm where quantitative data meets qualitative judgment, allowing venture capitalists to balance their ‘gut feelings’ with empirical evidence. This harmonious blend of technology and human intuition paves the way for more informed and strategic investment decisions, ultimately enhancing the potential for outsized returns.
The Future of AI in VC
As AI and ML technologies continue to evolve, their impact on venture capital is expected to grow even more profound. Future advancements could lead to more sophisticated predictive analytics, enabling VCs to identify emerging trends and investment opportunities with even greater accuracy. Additionally, AI could automate more of the due diligence process, analyzing a startup’s potential for success at an unprecedented scale and speed. The integration of AI in VC could also lead to more personalized investment strategies, where algorithms tailor recommendations based on a firm’s specific goals and risk tolerance.
Lessons from the Field
Insights from experienced venture capitalists reveal the importance of blending data-driven strategies with human intuition. Despite the significant advantages that AI and ML offer, seasoned VCs emphasize that successful investing still requires a human touch. Instincts, experience, and personal relationships play a crucial role in navigating the complexities of startup investing. For instance, understanding a founder’s vision and drive can be just as important as the startup’s data metrics when making an investment decision.
Veteran investors caution against over-reliance on data alone, noting that while data can guide and inform decisions, it cannot capture the full spectrum of factors that contribute to a startup’s success. The art of VC investing, therefore, lies in the ability to marry objective data analysis with subjective judgment, leveraging the strengths of both to optimize investment outcomes.
Future Outlook and Emerging Trends
Adapting to the Data-Driven Future
The venture capital landscape is inexorably moving towards a more data-centric approach, a trend fueled by the exponential growth of AI and machine learning technologies. This shift heralds a new era where decision-making processes are increasingly informed by vast datasets, predictive analytics, and automated systems. For VC firms, staying ahead in the technology adoption curve is not just advantageous; it’s imperative. This involves not only leveraging the latest in data analytics but also fostering a culture of continuous learning and adaptability. As the data technologies evolve, so too must the strategies and frameworks within which VCs operate, ensuring they can efficiently identify and capitalize on emerging opportunities.
Emerging Sectors and Technologies
In the future, data-driven venture capital is poised to make a significant impact across various sectors, notably healthcare technology, green technologies, and blockchain. In healthcare tech, data analytics can revolutionize personalized medicine, diagnostic processes, and patient care models. Green technologies stand to benefit from data-driven insights into energy consumption patterns, efficiency improvements, and sustainable practices. Meanwhile, blockchain technology, with its emphasis on transparency and security, offers fertile ground for data analytics to optimize financial transactions, supply chain management, and digital identities. These sectors represent just the tip of the iceberg, with data-driven VC strategies likely to unlock unprecedented value and innovation.
FAQs: Demystifying Data in VC
What data sources are most valuable for VCs?
VCs utilize a wide array of data sources, including public web data, financial records, market analyses, and satellite imagery, to gather comprehensive insights into potential investments.
What tools and techniques are essential for data-driven VC?
Essential tools include AI and ML algorithms for predictive analytics, data visualization software for interpreting complex datasets, and platforms that facilitate efficient data management and analysis.
Are there ethical considerations in using data for VC investments?
Yes, ethical considerations revolve around data privacy, the potential for bias in algorithmic decision-making, and the need for transparency in how data informs investment decisions.
Conclusion: The Integral Role of Data in Shaping VC’s Future
The integration of data analytics into venture capital marks a pivotal shift towards a more nuanced, efficient, and predictive approach to investment decision-making. This evolution underscores the vital balance between leveraging data-driven insights and retaining the indispensable element of human intuition that has long guided the VC industry. As technologies continue to advance, the ability of venture capital firms to adapt, innovate, and incorporate data into their strategic frameworks will be paramount. This adaptability not only positions VCs to capitalize on the next wave of groundbreaking startups but also ensures their continued relevance in an increasingly data-driven world.